
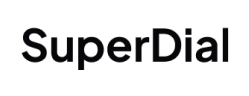

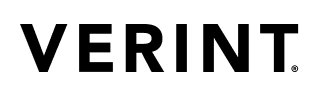




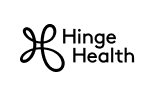
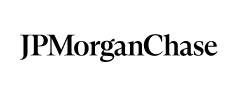

Achieve data privacy and AI optimization
In AI model training
Retain your data’s richness and preserve its statistics by replacing PII with synthetic values, to ensure optimal model training for LLM fine-tuning and custom models.
In RAG systems
Provide LLMs redacted data while optionally exposing the unredacted text to approved users. Automate pipelines to extract and normalize unstructured data into AI-ready formats.
In LLM workflows
Redact sensitive information prior to using it within LLM prompts to prevent sensitive values from ever entering the chatbot system.
In your lower environments
Accelerate data science based development with realistic test data that ensures data utility and data privacy throughout your lower environments
See Textual protect your data in real-time
Industry-leading sensitive data detection, redaction, and synthesis
Input
Connect Textual to your data store or upload files in any format via an intuitive UI or by feeding text directly into the Textual SDK.
Extract
Automatically extract your free-text data and detect over thirty sensitive entity types with Textual’s multilingual NER models.

Protect
Leverage granular controls to de-identify your data consistently, either through redaction or realistic synthesis, replacing sensitive values while maintaining semantic integrity.
Optionally certify that PHI data de-identification is HIPAA-compliant through our partnership with an expert determination provider.
Deliver
Output your protected data in its original file format or in a standardized, markdown format optimized for model training and RAG systems.
Support for all your data formats
Accessible where your data lives
Deploy Textual seamlessly into your own cloud environment through native integrations with cloud object stores, including S3, GCS, and Azure Blob Storage, or leverage our cloud-hosted service.
Available through your cloud provider
Burn down your cloud commitments by procuring Textual via the Snowflake Marketplace, AWS Marketplace, and Google Cloud Marketplace.
Secure your sensitive free-text data with Tonic Textual.
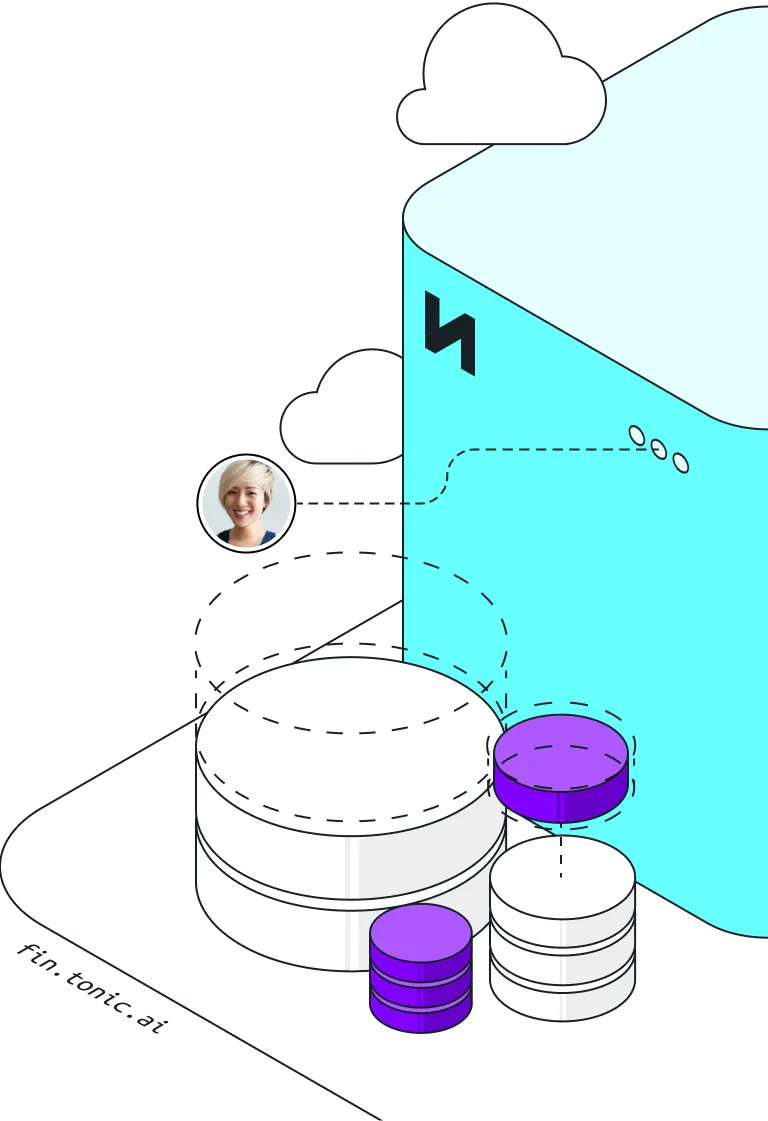