As generative AI models become increasingly integrated into our daily lives, the importance of embedding privacy and security at the core of any AI model becomes ever more critical. Ensuring that these systems are both secure and trustworthy is essential to protecting sensitive information and maintaining public trust. This ground-up approach to privacy and security, known as "Privacy by Design," is the cornerstone for the sustainable, safe growth of generative AI tools.
Generative AI privacy risks
As more organizations integrate generative AI models into their operations, understanding and mitigating the associated privacy risks is critical. These concerns are particularly significant in industries that handle sensitive data, such as finance or health care. Here are a few generative AI privacy risks to be aware of:
- Collection of sensitive data
- Data exfiltration
- Model inversion, re-identification, and data leakage
- Ethical and regulatory implications
- Automated decisions and bias
- Transparency and accountability in AI operations
Collection of sensitive data
Generative AI systems often require extensive training datasets, which can include highly sensitive personal, financial, or business information. Handling this much confidential data raises significant generative AI privacy risks, calling for stringent security measures and compliance with data protection regulations.
Data exfiltration
This risk involves the unauthorized access and theft of sensitive data processed by AI systems, which can pose a significant threat in sectors where data security is paramount. Protecting against data exfiltration requires robust security protocols and constant attention.
Model inversion, re-identification, and data leakage
AI models can potentially be exploited to extract or reconstruct personally identifiable information (PII), effectively undoing anonymization efforts. This generative AI privacy risk is particularly acute in environments where it is critical to maintain data confidentiality, requiring advanced measures to prevent data leakage.
Ethical and regulatory implications
There is a significant challenge posed by navigating the complex landscape of ethical norms and regulatory requirements around generative AI. Generative AI must adhere to a myriad of regulations designed to protect data privacy, which means compliance must be a constant effort for developers and businesses alike.
Automated decisions and bias
AI models trained on biased datasets can lead to unethical or unfair automated decisions, both of which affect customer trust and compliance with legal standards. Mitigating this risk involves careful design and continuous monitoring of AI systems to ensure fairness and transparency.
Transparency and accountability in AI operations
Ensuring that AI processes are transparent and accountable is key to maintaining regulatory compliance and user trust. This involves clear documentation of AI decision-making processes and being able to explain and justify them in understandable terms.
Understanding Privacy by Design
Privacy by Design, or Privacy by Default, is a framework that embeds privacy into technology design from the start. By building in generative AI privacy from the outset and then ensuring end-to-end security throughout the data lifecycle, this framework takes a more proactive approach to data security. Organizations can enhance user trust and comply more easily with regulations such as GDPR––in fact, Privacy by Design is a core tenet of GDPR––often leading to significant cost savings.
Privacy by Design is built upon seven foundational principles that guide the secure and private development of technologies:
- Proactive not reactive; preventative not remedial: This principle emphasizes anticipating and preventing privacy invasions before they happen, rather than reacting after the fact.
- Privacy as the default setting: Systems and business practices are designed to automatically protect privacy, without requiring individual intervention.
- Privacy embedded into design: Privacy is integrated directly into the design and architecture of IT systems and business practices, ensuring it is not an afterthought.
- End-to-end security – full lifecycle protection: This tenet ensures that data is securely protected throughout its entire lifecycle, from initial collection to final deletion.
Incorporating Privacy by Design principles can significantly boost consumer trust and brand preference. A study conducted by Google and Ipsos highlighted that a positive privacy experience can increase share of brand preference by as much as 49%. Additionally, while an overwhelming 76% of participants expressed a desire for control over the data they share with companies, only 11% felt they actually had complete control.
This gap underscores the critical need for businesses to adopt proactive privacy measures that are embedded by design. By doing so, they can enhance user trust, comply with privacy regulations, and drive consumer engagement and loyalty.
Unblock your AI initiatives and build features faster by securely leveraging your free-text data.
How to implement privacy by design in generative AI
Here’s how to apply the principles of Privacy in Design to your generative AI model:
Minimizing data collection and retention
To ensure the privacy of generative AI systems, it is critical to restrict the collection and retention of data. By collecting only the essential data required for the functionality of generative AI models and implementing strict data retention policies, organizations can significantly reduce privacy risks.
Transparency and explainability
It's important that generative AI systems operate transparently, with mechanisms that allow users to understand how their data is used and how decisions are made. Implementing explainable AI practices helps demystify the operations of generative AI, making them understandable to non-experts and thereby bolstering trust and compliance.
Implementing access control and user consent
Privacy by Design also involves robust access control and informed user consent mechanisms. Access to sensitive information should be strictly managed, with defined roles and permissions, while user consent for data collection and processing should be obtained through clear, straightforward communication to ensure that users are fully aware of how their data is being used.
Integrating data de-identification and data synthesis into model training workflows
Integrating data de-identification techniques in the training datasets for generative AI can safeguard privacy by removing or masking personally identifiable information. Additionally, using data synthesis for generating training data can further minimize the risk of exposing sensitive information, allowing generative AI models to learn from data that is not correlated with real individuals.
Leveraging differential privacy and federated learning
Differential privacy introduces randomness into datasets, making it difficult to identify individual data points––crucial when training generative AI models on sensitive information. Federated learning, on the other hand, allows for the training of models on decentralized data, ensuring that the actual datasets do not leave their original location, maintaining privacy across AI systems.
Continuous monitoring, auditing, and risk assessments
Continuous monitoring and regular audits are necessary for maintaining the integrity of privacy practices. These help ensure that all aspects of AI systems are consistently compliant with privacy laws and that any potential vulnerabilities are identified and addressed promptly. Regular risk assessments can evaluate how data processing impacts privacy, helping mitigate risks before they materialize.
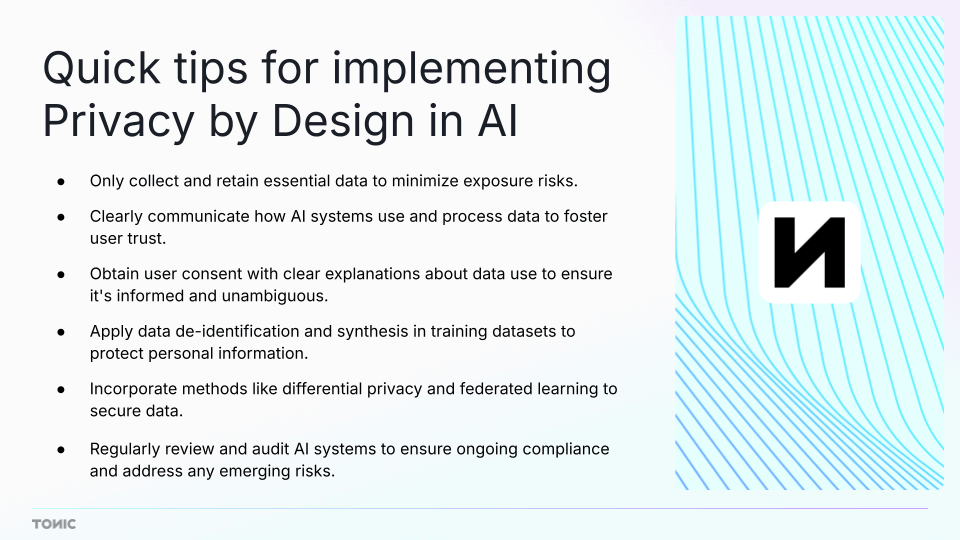
Future landscape of privacy-conscious generative AI
As generative AI continues to advance, the integration of robust privacy protections becomes more and more crucial. Technologies like homomorphic encryption––which allows data to be processed while still encrypted––are setting the stage for advanced privacy in AI operations. These technologies ensure that sensitive information remains protected, fostering trust and compliance with evolving privacy standards.
The role of policymakers is also pivotal. They are challenged to develop generative AI privacy regulations that protect privacy without hindering technological innovation. By crafting flexible, adaptive policies, they can ensure that privacy standards keep pace with technological developments.
And, for developers and organizations alike, adopting Privacy by Design principles is essential. This approach not only aligns with current regulations but also prepares AI systems for future legislative changes, minimizing compliance risks and positioning companies as leaders in ethical AI development.
Conclusion
As we look to the future, the integration of robust generative AI privacy measures will be crucial in advancing generative AI. It is imperative for all stakeholders—developers, organizations, and policymakers—to continue educating themselves about secure AI systems and remain informed about the ever-changing regulations and trends. By doing so, they can ensure that AI technologies not only deliver exceptional capabilities but also respect and protect the privacy of users.
Let this be a call to action: integrate Privacy by Design into your AI development to safeguard against potential regulations and build systems that users can trust and rely on. Use this infrastructure so that your company and its generative AI models will stay informed, stay compliant, and lead the way in ethical AI development. Connect with our team to learn more about how Tonic.ai is enabling and advancing Privacy by Design.